The Hydrolab Group, coordinated by Prof. Salvatore Manfreda, is engaged in the development of advanced methodologies for monitoring water quality in riverine and lake ecosystems. The core activities of the research group focus on low-cost, image-based techniques and the integration of remote sensing and machine learning approaches aimed at overcoming the spatial, temporal, and cost limitations of traditional in-situ water quality monitoring methods, as shown in Figure 1.
Through scalable, transferable, and citizen-driven solutions, this works enhance environmental monitoring practices and support sustainable water resources management.
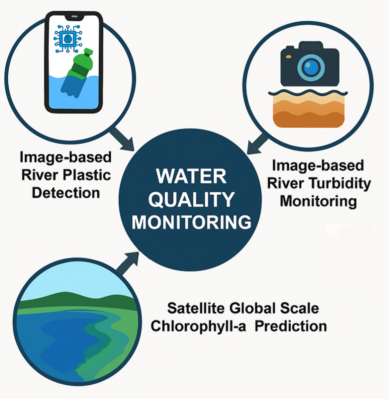
Figure 1. Overview of Hydrolab’s core activities in water quality monitoring: plastic detection, turbidity monitoring, and satellite-based chlorophyll-a prediction.
RESEARCH ACTIVITIES
Image-Based River Plastic Detection
Macroplastics represent one of the most pressing forms of pollution in riverine environments. Detecting and quantifying them remains a challenge due to spatial variability and the lack of standardized, scalable monitoring systems. To address these limitations, recent studies of Saddi et al.(2025) have focused on developing image-based systems for the detection of floating plastics using RGB images acquired via smartphones, fixed cameras, and UAVs. These images are analyzed using deep learning algorithms, specifically YOLOv7 and YOLOv8, to classify multiple categories of litter across varied environmental contexts.
Particular attention is given to the creation of small but environmentally diverse datasets, which have proven more effective for model transferability than larger homogeneous datasets. First results indicate that diverse datasets significantly improve generalization and scalability, enabling the deployment of detection systems across geographically distinct river systems. This approach paves the way for real-time, mobile-based plastic monitoring solutions and supports future integration into citizen science initiatives.
Image-Based River Turbidity Monitoring
Turbidity is a key indicator of water quality, reflecting the presence of suspended solids, organic matter, and floating pollutants. Conventional measurement devices, such as turbidity sensors and probes, are often limited in their spatial and temporal resolution and are costly to implement on a large scale. To overcome these constraints, a new image analysis framework has been developed by Miglino et al. (2024), utilizing different camera systems, including fixed trap cameras, UAVs, and multispectral sensors.
Field experiments involving artificially induced turbidity events have demonstrated that single-band pixel intensity values from digital images are reliable proxies for short-term turbidity assessment, when supported by a robust image processing procedure. This technique allows for continuous, high-frequency data acquisition using affordable and easily deployable equipment. Several campaigns under varying hydrological and environmental riverine conditions validated the method. The framework is also being adapted for use in mobile applications, which will allow citizens to contribute observational data, enhancing monitoring coverage at basin scale, enabling early detection of turbidity events.
Satellite-Based Chlorophyll-a Prediction at Global Scale
Chlorophyll-a is a fundamental proxy for assessing phytoplankton biomass and the risk of eutrophication in both inland and coastal waters. Traditional in-situ sampling techniques are time-consuming and spatially limited. To address this, a machine learning-based framework has been developed that utilizes satellite imagery to predict chlorophyll-a concentrations at a global scale.
The framework of Moe et al.(2025) focuses on data quality, analyzing two groups of input features: simple band reflectance values and mathematically derived transformations. Multiple machine learning models have been tested for their predictive performance and ability to generalize across diverse environments. The system has shown reliable spatial-temporal consistency, particularly in external validation scenarios, demonstrating its transferability to unobserved locations. This method offers a robust and scalable tool for the continuous monitoring of eutrophication and supports decision-making processes in water ecosystems management and environmental policy.
Related Projects:
OurMED – Sustainable Water Storage and Distribution in the Mediterranean
This initiative is part of the PRIMA Programme supported by the European Union’s Horizon 2020 Research and Innovation Programme. It aims to foster innovative approaches to water storage, distribution, and monitoring, tailored to the unique environmental and societal challenges of the Mediterranean basin. Hydrolab contributes with scalable Earth Observation and in-field monitoring techniques.
RiverWatch – A Citizen Science Approach to River Pollution Monitoring
Funded by the Italian Ministry of University and Research (PRIN 2022), RiverWatch promotes active public engagement in monitoring river health. Through image-based techniques and mobile app development, the project focuses on tracking macroplastics and turbidity using community-collected data. The Sarno River serves as a flagship site for testing and validating this participatory monitoring system.
References:
Manfreda, S., D. Miglino, K. C. Saddi, S. Jomaa, A. Etner, M. Perks, D. Strelnikova, S. Peña-Haro, I. Maddock, F. Tauro, S. Grimaldi, Y. Zeng, G. Gonçalves, T. Bogaard, T. van Emmerik, M. Bussettini, S. Mariani, G. Marchetti, B. Lastoria, B. Su, M. Rode, Advancing river monitoring using image-based techniques: challenges and opportunities, Hydrological Sciences Journal, Pages 657-677, (doi: 10.1080/02626667.2024.2333846) 2024. [pdf]
Marye, A.T., C. Caramiello, D. De Nardi, D. Miglino, G. Proietti, K. Cathleen Saddi, C. Biscarini, S. Manfreda, M. Poggi, F. Tauro, Remote Sensing for Monitoring Macroplastics in Rivers: A Review, WIREs Water, Volume12, Issue2, 2025. [pdf]
Miglino, D., Saddi, K. C., Isgrò, F., Jomaa, S., Rode, M., and Manfreda, S.: Technical note: Image processing for continuous river turbidity monitoring – full scale tests and potential applications, EGUsphere [preprint], 2024. [pdf]
Saddi, K. C., Miglino, D., Isgro, F., Van Emmerik, T. H., & Manfreda, S. (2024, July). Balancing Accuracy and Efficiency for River Plastic Monitoring. In IGARSS 2024-2024 IEEE International Geoscience and Remote Sensing Symposium (pp. 3836-3840). IEEE.
Miglino, D., S. Jomaa, M. Rode, F. Isgro, S.Manfreda, Monitoring Water Turbidity Using Remote Sensing Techniques. Environ. Sci. Proc., 21, 63, 2022. [pdf]